The Key Risks and Dangers of Artificial Intelligence
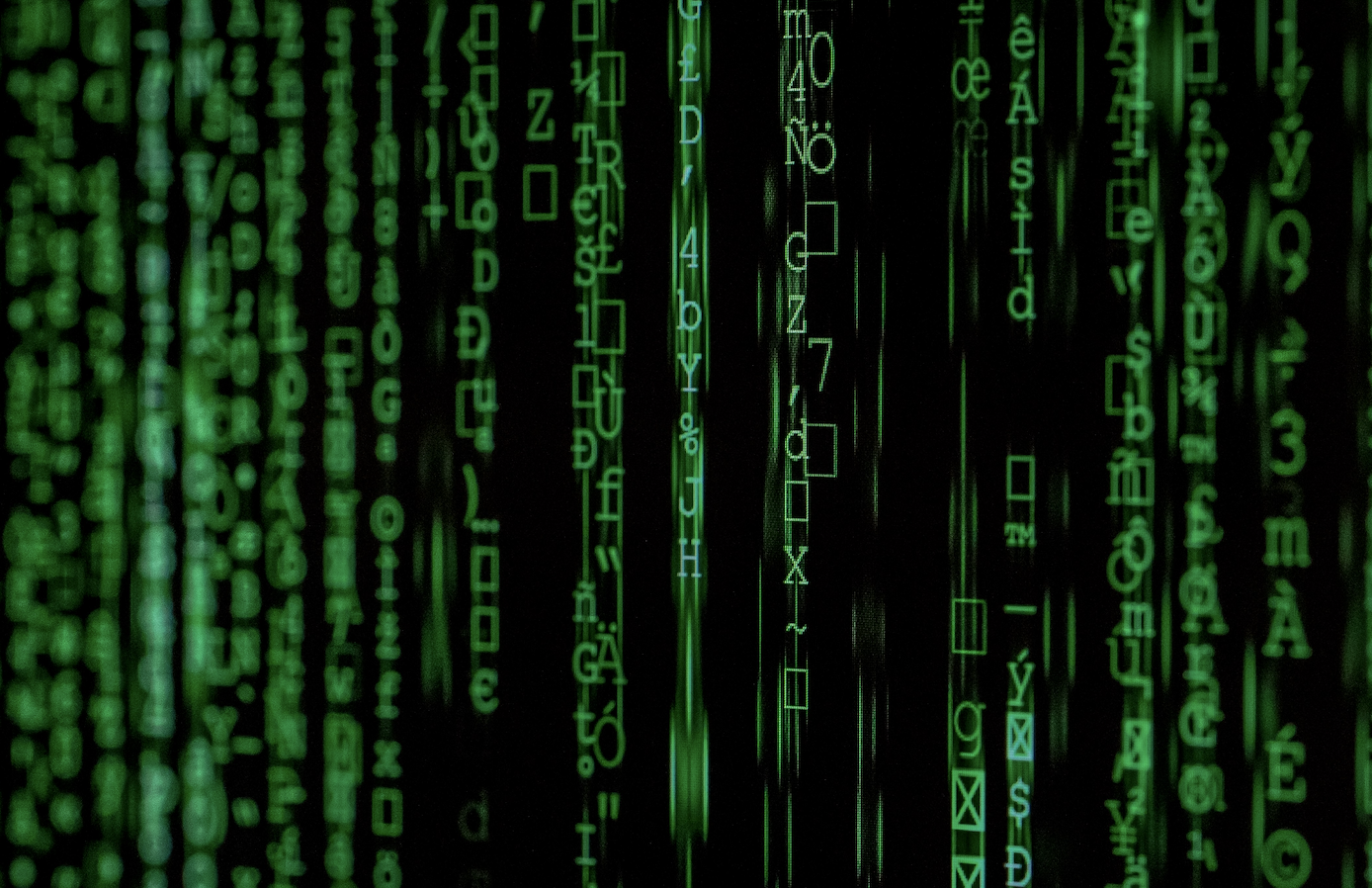
Artificial Intelligence (AI) has seen rapid progress in the last few years that few considered possible. While it is still in its early stages of being implemented in many organisations, experts caution that AI applications can pose a threat to fundamental human rights, democracy, and the environment. We have already seen an array of examples where AI violated user’s data privacy, abused biometric data, or discriminated against people due to biases embedded in training data leading to “algorithmic bias” - and this is only the beginning.
This article sketches the key risk sources and ethical AI concerns around developing and deploying AI systems.
The Complex Nature of Artificial Intelligence Failures
Before discussing how AI may ethically fail us, it is useful to understand what makes AI technology risky in itself: its complexity and unpredictability. To get a grasp on where those risks come from, we briefly explain the two main techniques currently used in the AI field: Machine Learning and Deep Learning.
What is Machine Learning?
Machine learning, a key branch of AI, finds widespread adoption across industries for its predictive capabilities, with applications ranging from forecasting product demand to personalising recommendations. In essence, machine learning seeks to identify patterns in existing data to make predictions about new data. The main characteristics of machine learning are:
- Data-driven learning: Unlike traditional programs, machine learning systems don't require explicit instructions for every task. Instead, they learn from examples, improving their performance over time. This approach enables them to tackle problems that are difficult to articulate.
- Probabilistic or stochastic nature: Machine learning systems identify patterns and relationships in data to make predictions. As a result, their outputs are - in essence - educated guesses. This means they can produce errors or reproduce biases in data, offering the most probable outcome based on learned patterns, which may not always align with the actual truth.
What is Deep Learning?
Deep learning is a class of algorithms within ML that has powered the bulk of current innovations and progress of AI in the last decade, from large language models to face recognition systems, voice assistants to medical diagnostics. The main characteristics of deep learning are:
- Complex architecture: Inspired by the structure of the human brain, deep learning uses layers of artificial neurons - simple computing units that process and pass on information. The term 'deep' refers to the multiple layers in these networks, which allow them to model complex relationships in data.
- Opaque decision-making: While deep learning systems can identify intricate data patterns, understanding how they reach specific conclusions is challenging. This lack of ‘explainability’ poses difficulties even for the data scientists who develop these systems, making it hard to fully explain the reasoning behind particular outputs.
How does the Nature of AI Translate into Risks?
While machine learning and deep learning offer powerful capabilities, their core characteristics can also introduce specific risks and dangers. Let's examine how these features can lead to potential issues in AI systems.
Risks Arising from its Architecture
- Challenges in AI integration: Small mistakes in early layers can compound through the system, potentially resulting in significant errors in the final output. Furthermore, adjusting one part of the system may have unforeseen effects on other parts due to the interconnected nature of the layers.
Example: The integration of AI in complex systems, such as autonomous vehicles, presents significant challenges due to the shift of human error from operational actions to coding errors (see IEEE spectrum). Autonomous vehicles, for instance, rely heavily on software that is prone to faults, leading to unpredictable failures like crashes and phantom braking. AI models struggle to make judgments under uncertainty, especially in dynamic environments where their training data may not cover all scenarios, leading to errors that are difficult to foresee and mitigate. Maintaining these AI systems is critical, as models require constant updates to remain effective and relevant.
- Environmental harm: Training large and complex AI systems poses a serious risk to international efforts against climate change and pollution.
Example: It is estimated that ChatGPT alone consumes over half a million kilowatt of electricity a day. Developing and running AI systems already has a sizable carbon footprint and we have only just begun. To meet increased demands for AI systems in the future, we may need to build more servers, potentially taking away from natural habitats, diminishing and polluting water supplies, and exacerbating air pollution.
Risks Arising from Data-Driven Learning
- Bias amplification and discrimination: If training data contains societal biases, AI systems may perpetuate or even exacerbate these biases in their outputs.
Example: Amazon developed an AI tool to evaluate job applications, but it inadvertently learned to discriminate against women. The system was trained on the company's historical hiring data, which reflected the tech industry's long-standing male dominance. As a result, the AI began to favour male applicants, downgrading CVs that included terms associated with women, such as “women's chess club”.
- Vulnerability to data poisoning: Malicious actors could potentially manipulate training data to induce harmful behaviours in AI systems.
Example: An article in the New York Times explores the potential for AI chatbots to spread disinformation from data poisoning, ahead of the U.S. presidential election. By customising chatbots using social media data from Reddit and Parler, the study shows how easily AI can adopt partisan viewpoints and generate biassed or inflammatory responses. This highlights the risk of AI tools being manipulated to flood social media with misleading content.
- Malicious use: Scammers, terrorists, and power-seeking individuals could potentially manipulate training data to induce harmful behaviours in AI systems.
Example: As digital financial transactions become more prevalent, cybercriminals are leveraging AI to develop increasingly sophisticated attack methods. Deepfake technology, powered by machine learning, allows fraudsters to create convincingly realistic synthetic media for impersonation, as exemplified by a $25 million fraud case involving deep fake video calls. AI-powered password cracking tools, such as PassGAN, have shown alarming efficiency, with 51% of passwords cracked in less than a minute. The surge in data breaches, exacerbated by AI, has led to a wealth of stolen identity credentials available to criminals. This has resulted in an increase in synthetic identity fraud, exposing lenders to potential losses of $3.1 billion
Risks of Probabilistic Outputs
- Mis- and disinformation: Users may over-trust AI outputs, not realising they're based on probabilities rather than certainties, also referred to as hallucinations.
Example: A study by Democracy Reporting International assessed how tech companies adapted their chatbots after being alerted to electoral misinformation issues. Despite some changes, the study found significant ongoing problems with how these chatbots handled electoral content across different EU languages. A key concern was that openAI's ChatGPT models rarely refused to answer electoral questions and continued to provide many incorrect responses, indicating a concerning level of disinformation.
- Values-unaware results: AI systems may produce outputs that violate social norms or ethics, such as chatbots promoting hate speech or suggesting harmful actions. This occurs because the system optimises for statistical patterns in data without an inherent understanding of wider societal values or moral considerations.
Example: Gab, a far-right social network, has launched Gab AI, a platform featuring nearly 100 chatbots, including controversial figures like Adolf Hitler, Donald Trump, and Ted Kaczynski, some of which deny the Holocaust and spread conspiracy theories (Read full article in WIRED). The chatbots, including a default bot named Arya, promote narratives questioning the Holocaust, rejecting vaccines, and claiming that the 2020 U.S. election was rigged. Experts warn that these chatbots could normalise disinformation and radicalise users by creating echo chambers of extremist beliefs. Gab's founder, Andrew Torba, defends the platform as “unbiased,” allowing all views, including those that dispute mainstream narratives.
Risks of Opaque Decision-Making and Lack of Explainability
- Regulatory challenges: It becomes difficult to ensure AI systems comply with laws and ethical guidelines when their decision-making process is not fully understood or “explainable”.
Example: The European Commission's investigation into TikTok's algorithm illustrates this challenge. In February 2024, the EC launched a probe into TikTok's addictive design and opaque AI systems, particularly concerning child safety. Regulators struggled to assess whether TikTok's recommendation algorithm complied with the Digital Services Act, as the company's AI decision-making processes were not transparent. This opacity made it challenging to determine if the platform was adequately protecting minors from harmful content or respecting user choice in content recommendations. The case highlights how the 'black box' nature of advanced AI systems can impede effective regulation and enforcement of digital safety laws.
- Limited accountability: When AI systems make mistakes, it can be challenging to determine why they occurred,how to prevent them in the future, and who bears responsibility for those mistakes
Example: A lawyer in New York used ChatGPT to prepare a court filing, which included citations of non-existent legal cases. When challenged, the lawyer claimed he was unaware of ChatGPT's potential to generate false information, despite having practised law for 30 years. This case underscores the risks of blindly relying on AI tools in professional settings, particularly in law where accuracy is paramount. It raises questions about who bears responsibility when AI generates false information - the lawyer who used it without verification, or the AI developers?
The Wider Social Concerns of AI
AI has serious potential to violate fundamental rights and infringe upon our ethical norms and social values if AI technologies remain unregulated, incentivising an AI race on the market eschewing safety and ethics for short term gains. The main risks resulting from unsafe development in the AI sector are:
- Fundamental Rights Violations: AI systems can significantly impact fundamental rights, particularly privacy and data protection. The widespread collection and processing of personal data for AI training often occurs without adequate consent, violating individuals' right to privacy. For instance, the Swedish Data Protection Authority fined a school in 2019 for using facial recognition to track student attendance, breaching GDPR and students' privacy rights. Similarly, the use of AI in predictive policing, such as the now-discontinued HART (Harm Assessment Risk Tool) in Durham, UK, raised concerns about potential bias and infringement of the right to non-discrimination.
- Job Displacement: Job displacement remains a significant concern, with AI's rapid advancement threatening to automate many roles. For example, JPMorgan Chase's COIN software now performs document review tasks in seconds that previously took lawyers 360,000 hours annually. Similarly, AI writing assistants are increasingly used in journalism, with outlets like Associated Press using AI to generate thousands of earnings reports. While AI enhances productivity in these fields, it also raises concerns about the future of human employment in such sectors, as AI systems don't require salaries, maternity leave, or labour rights, making them potentially more cost-effective for employers in the long run.
The 3 Key Risks of AI for Organisations
Considering the vast risk potential of AI, organisations developing and employing AI are responsible to ensure a robust safety culture and ethical soundness of their system. With the rise of AI legislation globally, disregarding the risks of AI can have direct consequences for organisations.
- Legal Risks: Organisations may face considerable costs for legal liabilities should their AI system violate regulation or become part of lawsuits and reparations claims for consumer-related failures.
- Financial Risks: Organisations that fail to comply with regulations like the EU AI Act may face fines between 35.000.000 and 7.500.000 Euros or a percentage of its annual turnover. These fines are summative to other regulatory penalties, such as those under GDPR, potentially leading to substantive costs.
- Reputational Risks: Organisations embroiled in lawsuits and scandals pertaining to ethical failure of their AI systems are likely to face considerable losses in reputation. This may lead to revenue loss, loss of shareholders and loss of customer trust.
Read our blog article about the true costs of non-compliance if you would like to zoom in further on how the dangers of AI are relevant to you.
In a Nutshell: Why is AI dangerous?
In essence, AI poses significant risks due to the inherent difficulty in accurately predicting its behaviour. This unpredictability makes it challenging to anticipate and plan for accidents and malfunctions. The root cause of these dangers lies partly in the nature of AI systems: they are data-driven, probabilistic, complex, and often opaque.
For most complex AI systems, developers have limited understanding of the exact inner workings of deep learning algorithms, which process data by creating artificial neural networks that closely mimic human neural networks in complexity. This lack of transparency complicates troubleshooting and safety analysis of certain systems, as it's often unclear how AI arrives at its outputs.
On a broader societal level, the primary concerns are the potential violations of fundamental rights, significant job displacement and environmental impacts. These risks underscore the need for careful development, deployment, and regulation of AI technologies to ensure they benefit society while minimising potential harm.
It is important for organisations to take the organisational and societal risks seriously. Ignorance and negligence of those risks pose serious legal, financial, and reputational risks.
More on AI regulation
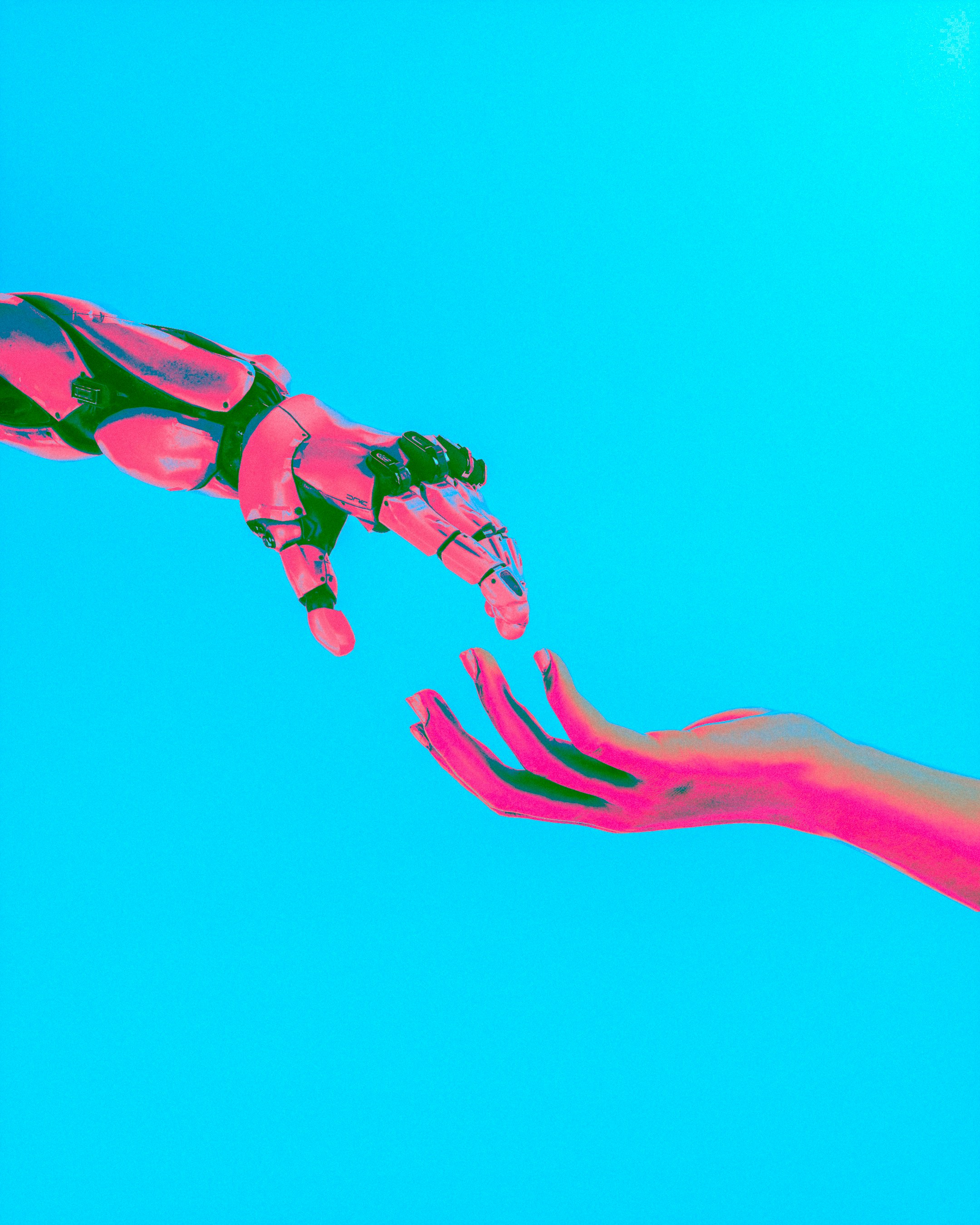
From AI Companions to AI Girlfriends: Is Black Mirror coming to real life?
